Collaborative research work on adversarial machine learning between SKKU and LUC awarded the best paper award in SVCC’24
A paper titled "Unmasking the Vulnerabilities of Deep Learning Models: A Multi-Dimensional Analysis of Adversarial Attacks and Defenses" has been awarded the Best Paper Award at the 5th Silicon Valley Cybersecurity Conference (SVCC) 2024.
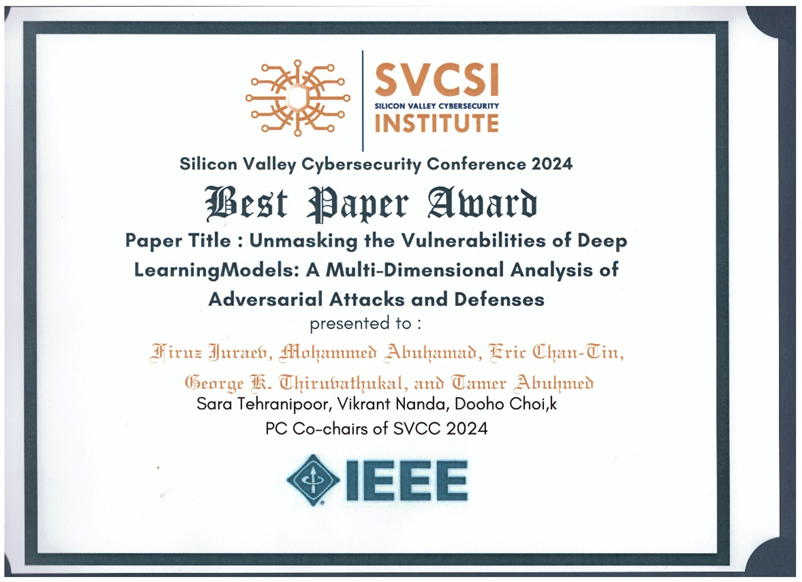
A paper titled "Unmasking the Vulnerabilities of Deep Learning Models: A Multi-Dimensional Analysis of Adversarial Attacks and Defenses" has been awarded the Best Paper Award at the 5th Silicon Valley Cybersecurity Conference (SVCC) 2024. This research is the result of an ongoing collaboration between Sungkyunkwan University and Loyola University Chicago, led by authors Firuz Juraev, Mohammed Abuhamad, Eric Chan-Tin, George K. Thiruvathukal, and Tamer Abuhmed. The study provides a comprehensive analysis of black-box adversarial attacks and defenses on deep learning (DL) models, highlighting the increased serious threat that can cause the DL model to misbehave and compromise the performance of critical applications that are using these vulnerable models.
This research addresses a critical aspect of deep learning by focusing on the robustness of models against adversarial attacks,said Dr. Sean Choi, session chair of SVCC 2024.Their findings provide invaluable insights that will help enhance the security and reliability of DL models in various safety-critical domains.
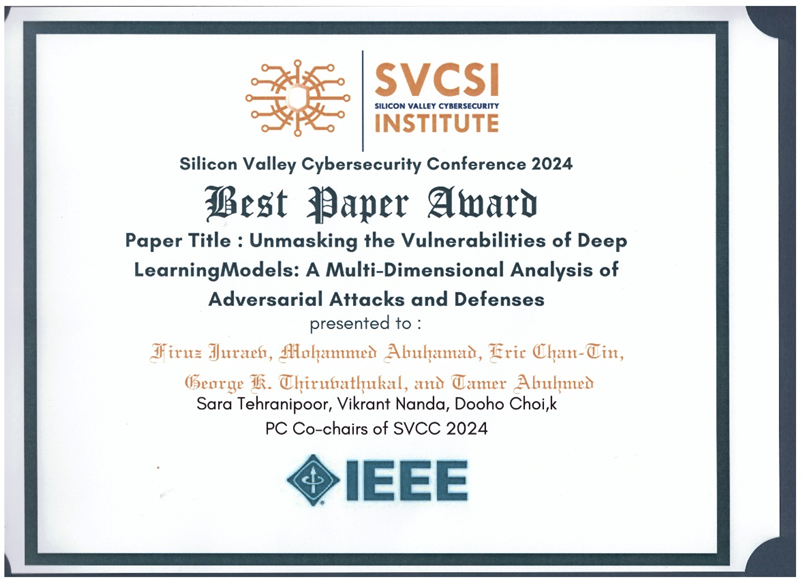
The paper's key contributions include a thorough investigation of various black-box attacks on diverse DL architectures. The authors demonstrate that while model complexity correlates with increased robustness, the number of model parameters alone does not ensure higher resilience to existing attacks. The research also explores how different components used in the model and training datasets impact model robustness, revealing significant insights for security-critical applications.
This award-winning research has significant real-world implications, particularly for enhancing the security and reliability of DL models in safety-critical domains like autonomous driving, healthcare and medical diagnosis, industrial automation, and surveillance. The findings emphasize the necessity of robust DL models that can withstand adversarial attacks, making it crucial to understand and defend against these threats. This study paves the way for future research on advanced attacks and the robustness of various DL architectures, including Vision Transformers, Graph Neural Networks, and Generative Adversarial Networks (GANs).